- People
-
Research
- Development in the Lifespan
- Quantitative Methods & Data Science
- Social Determinants of Health
- Innovation and Advancing Clinical Treatments
- Molecules, Neuronal Circuits and Behavior
- Theories and Applications in Social Psychology
- Faculty Publications
- Faculty Bookshelf
- Participate in Research at UVA Psychology
- Graduate
- Undergraduate
- Diversity
- Connect
Quantitative Methods & Data Science
Introduction
The University of Virginia Department of Psychology maintains a world-leading program in Quantitative Psychology. Our long-standing expertise in research methods, statistical analysis, and study design has established UVA as a pioneer in developing innovative quantitative approaches to psychological research.
Our faculty are internationally recognized leaders in mathematical, statistical, and data science methods. They contribute their expertise to virtually all research programs within the department while maintaining active collaborations with the UVA School of Data Science, School of Medicine, and School of Education and Human Development, and others. This interdisciplinary approach ensures our research remains at the cutting edge of quantitative methods in psychological science.
Our Faculty
- Steven Boker: Professor of Psychology; UVA 2024 Distinguished Researcher Award; 2019: Sells Award for Distinguished Multivariate Research from the Society for Multivariate Experimental Psychology
- Hudson Golino: Associate Professor of Psychology; UVA 2024 Excellence in Research Award
- Teague Rhine Henry: Assistant Professor of Psychology and Data Science
- M. Joseph Meyer: Assistant Professor of Psychology
- Cynthia Tong: Associate Professor of Psychology; 2024 All-University Teaching Award
- John Darrell Van Horn: Professor of Psychology and Data Science
- Karen Schmidt: Professor of Psychology
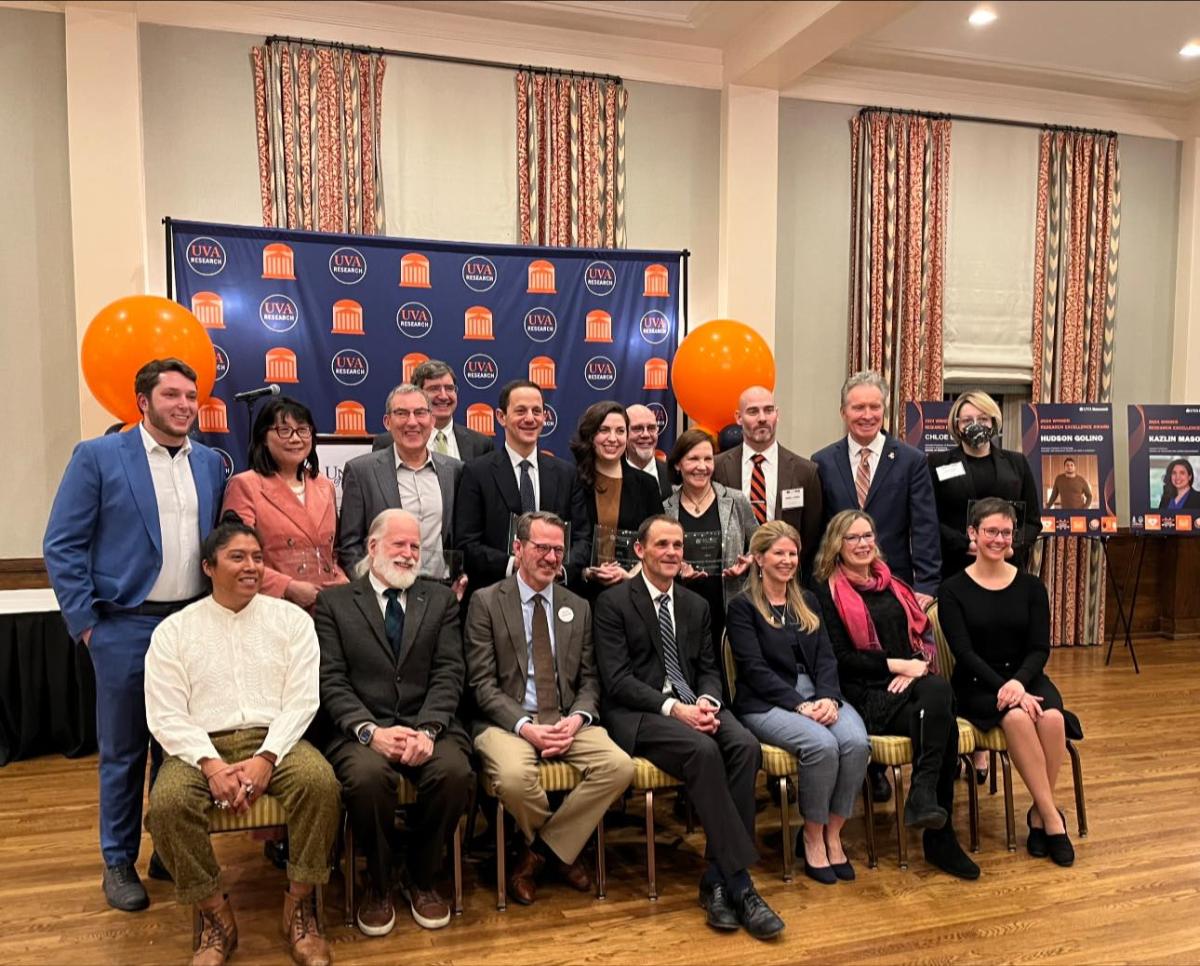
Our History
The program was founded by Dr. John R. Nesselroade (1936-2024), a prominent psychologist and methodologist who held the prestigious Hugh Scott Hamilton Professorship in Psychology at the University of Virginia. His groundbreaking work focused on lifespan developmental psychology and quantitative methods for studying intraindividual change and development over time. He was particularly renowned for his contributions to the study of intraindividual variability and development, pioneering methods for analyzing how individuals change across different time scales.
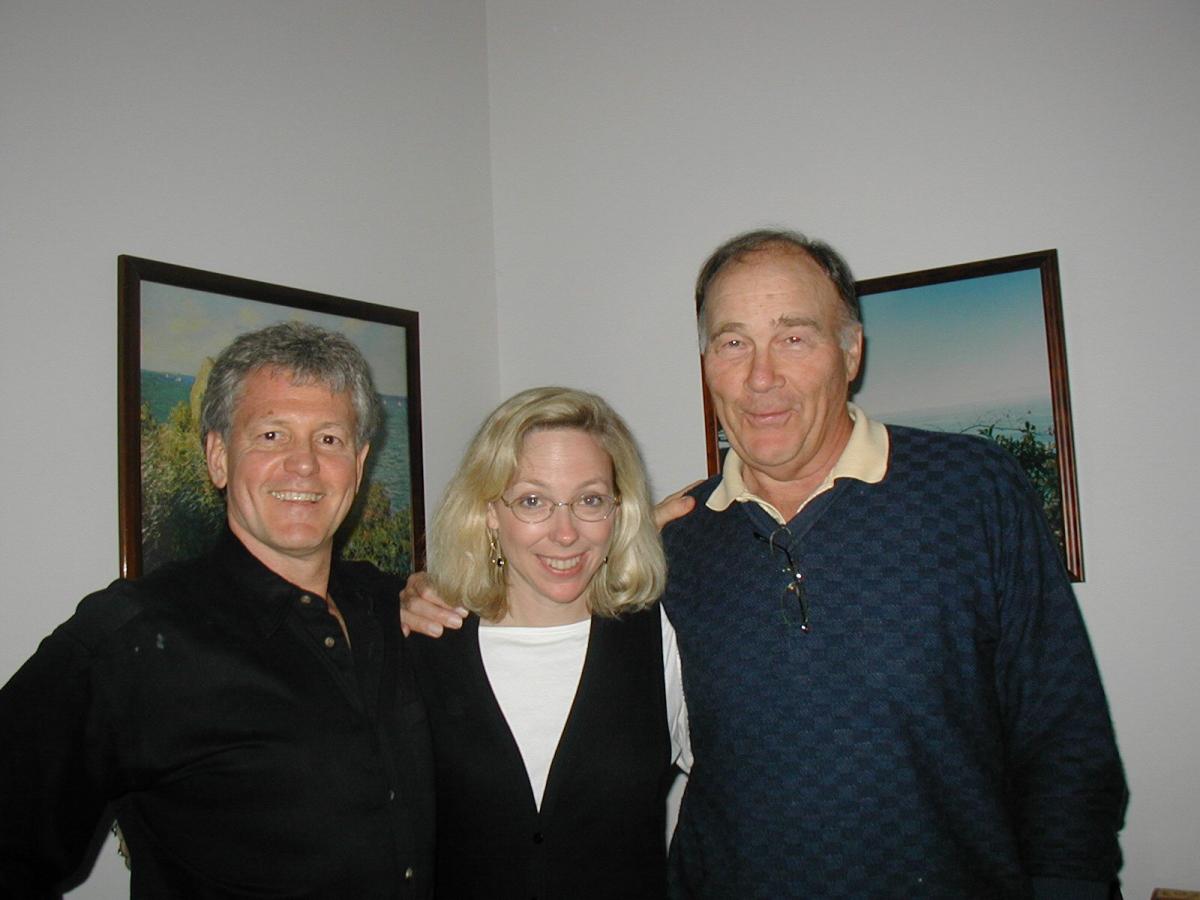
Throughout his distinguished career, Nesselroade made fundamental theoretical and methodological contributions to developmental psychology. He was a pioneer of the idiographic approach in psychological research, emphasizing the importance of studying individuals intensively over time rather than solely relying on group-level analyses. His collaborative work with Peter Molenaar on P-technique factor analysis and dynamic factor analysis revolutionized methods for studying psychological processes at the individual level. His leadership as president of the Society of Multivariate Experimental Psychology and numerous awards underscore his lasting impact on the field.
At the University of Virginia, Nesselroade, alongside influential figures such as Jack McArdle and Steven Boker, cultivated a powerful Quantitative Psychology program that has mentored generations of students. Many of these alumni have become leading quantitative psychology researchers at prestigious institutions worldwide, creating a lasting legacy in the field.
Current Areas of Research and Training
Structural Equation Modeling (SEM)
Structural equation modeling (SEM) is a powerful statistical technique to analyze complex relationships between observed and latent variables in psychological research. It allows researchers to test theoretical models about how different psychological constructs relate to one another, while accounting for measurement error and multiple simultaneous pathways of influence.
In psychology, SEM is particularly valuable for studying phenomena that cannot be directly observed, such as intelligence, depression, or motivation, by modeling these constructs through multiple observed indicators (like test scores or questionnaire responses). The technique is widely used in fields like personality psychology to understand how traits relate to behaviors, in developmental psychology to track changes over time, and in social psychology to model complex interpersonal processes.
Our program specializes in advancing SEM methodology through:
- Development of novel estimation methods for complex longitudinal data
- Integration of machine learning techniques with traditional SEM approaches
- Creation of tools for handling missing data and non-normal distributions
- Application of SEM to big data and high-dimensional problems
- Improving psychological measurement for inter and intraindividual analysis
Faculty: Steven Boker, M. Joseph Meyer, Cynthia Tong
Network Science
Graph theory in psychology provides a mathematical framework for analyzing and visualizing relationships between different psychological elements, whether they're symptoms, behaviors, neural connections, or social interactions. Our program leads innovative research in:
- Development of new network psychometric methods, such as Exploratory Graph Analysis, Dynamic Exploratory Graph Analysis, and related techniques
- Application of network analysis to psychological data
- Integration of temporal dynamics in psychological networks
- Advanced visualization techniques for complex psychological data
- New estimation methods
- Software Development
Our faculty pioneered several network-based approaches that have become standard tools in psychological research, particularly in the study of psychopathology and social relationships.
Faculty: Hudson Golino, Teague Rhine Henry
Psychometrics
Psychometrics is the field of study concerned with the theory and methodology of psychological measurement, which includes the design, development, and validation of measurement instruments and the analysis of measurement data. This discipline encompasses both the theoretical frameworks for how to measure psychological attributes (like intelligence, personality traits, or clinical symptoms) and the practical tools for doing so reliably and validly. Psychometricians develop and evaluate psychological tests, questionnaires, and other assessment tools using sophisticated statistical methods such as factor analysis, item response theory, and classical test theory. Their work is crucial across many areas of psychology - from educational testing (where psychometric principles guide the development of standardized tests) to clinical assessment (where accurate measurement of psychological symptoms is vital for diagnosis and treatment planning) to organizational psychology (where psychometric tools help in employee selection and performance evaluation). The field also addresses fundamental questions about the nature of psychological measurement itself, such as how to quantify abstract constructs, how to ensure measurements are consistent across different populations and contexts, and how to determine whether a measurement tool is actually measuring what it claims to measure.
Our psychometrics research focuses on advancing both theoretical frameworks and practical applications for psychological measurement. Key areas include:
- Modern test theory and item response modeling
- Computerized adaptive testing
- Network psychometric approaches to test construction
- Integration of machine learning with traditional psychometric methods
- Development of novel reliability and validity assessment techniques
We maintain strong connections with testing organizations and actively contribute to advancing measurement practices in educational, clinical, and organizational settings.
Faculty: Steven Boker, M. Joseph Meyer, Hudson Golino, Teague Rhine Henry, Karen Schmidt
Human Brain Mapping
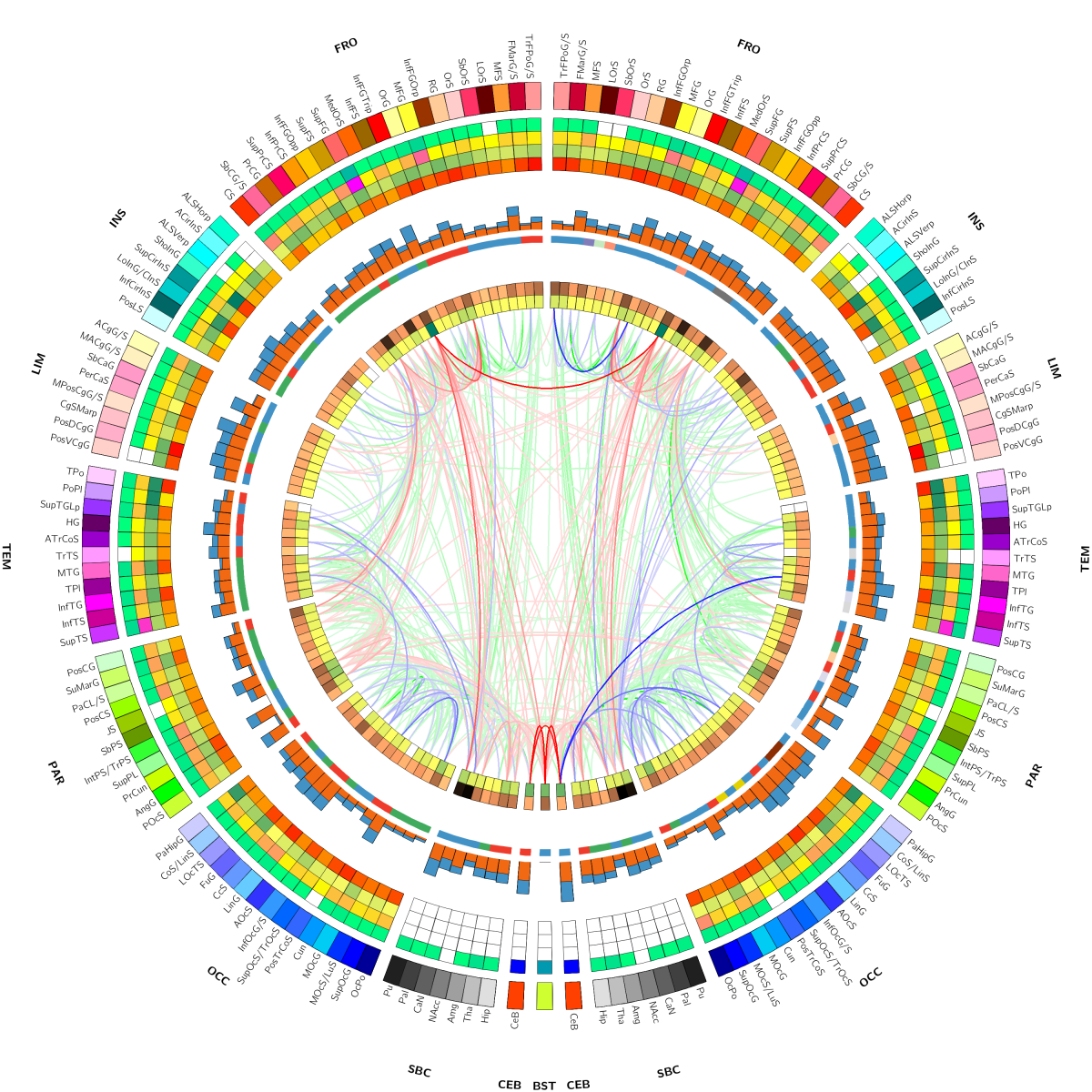
Brain imaging data analytics involves sophisticated computational methods to process, analyze, and interpret data from neuroimaging techniques like fMRI, EEG, and PET scans. This field combines elements of signal processing, statistical analysis, machine learning, and network theory to transform raw neuroimaging data into meaningful insights about brain structure and function. In psychology, these analytics enable researchers to study neural correlates of cognitive processes, emotional states, and behavioral patterns. For instance, researchers can examine how different brain regions activate and interact during specific tasks, track changes in brain connectivity associated with learning or development, and identify neural signatures of psychological conditions. Advanced techniques like multivariate pattern analysis (MVPA) and dynamic causal modeling (DCM) allow psychologists to decode mental states from brain activity patterns and understand causal relationships between brain regions. These analytical approaches have revolutionized our understanding of phenomena like decision-making, memory formation, emotional regulation, and various psychological disorders, bridging the gap between observable behavior and its underlying neural mechanisms.
Our program specializes in developing and applying advanced computational methods for analyzing neuroimaging data. Current research focuses on:
- Novel methods for processing and analyzing fMRI, EEG, and PET data
- Development of machine learning approaches for neuroimaging analysis
- Integration of network science with brain mapping
- Methods for analyzing brain connectivity and dynamics
- Tools for large-scale neuroimaging data management and analysis
Faculty: John Darrell Van Horn, Teague Rhine Henry
Non-linear Dynamics
Non-linear dynamics is the study of systems in which relationships between variables are not proportional, leading to complex behaviors such as feedback loops, chaos, and emergent phenomena. In psychology, non-linear dynamics is used to model and analyze processes that evolve over time in unpredictable or irregular ways, such as emotional regulation, cognitive development, or social interactions. By employing tools like differential equations, phase space analysis, and chaos theory, researchers can capture patterns in how psychological systems change under different conditions. Applications include understanding how individuals adapt to stress, modeling brain activity during cognition, and exploring group behaviors like decision-making or crowd dynamics. These approaches provide a more nuanced view of psychological phenomena, capturing the inherent complexity and variability of human behavior.
Our work in non-linear dynamics extends traditional statistical approaches to capture the complexity of psychological phenomena. Key areas include:
- Development of methods for analyzing psychological time series
- Application of dynamical systems theory to psychological processes
- Study of emergent phenomena in social and cognitive systems
- Integration of chaos theory with psychological measurement
- Advanced modeling of developmental processes
Faculty: Steven Boker
Large Language Models and Artificial Intelligence
Our program is at the forefront of integrating artificial intelligence and large language models (LLMs) into psychological research methods. This cutting-edge area focuses on:
- Development of AI-powered psychological assessment tools
- Validation of LLM-generated psychological instruments in silica
- Investigation of AI models' cognitive architectures
- Application of transformer models to psychological data analysis
- Integration of machine learning with traditional psychometric approaches
- Ethical considerations in AI-assisted psychological research
- Development of interpretable AI models for psychological applications
Our faculty leads innovative projects combining AI with quantitative psychology, including the development of AI-GENIE and other tools that leverage LLMs for psychological measurement. We also investigate how insights from cognitive psychology and psychometrics can inform the development of more robust and interpretable AI systems.
Faculty: Hudson Golino, Steven Boker
Software Packages Developed/Maintained by UVA Quantitative Psychology Faculty
Several software packages that are developed by our faculty are available for use:
The OpenMX Project
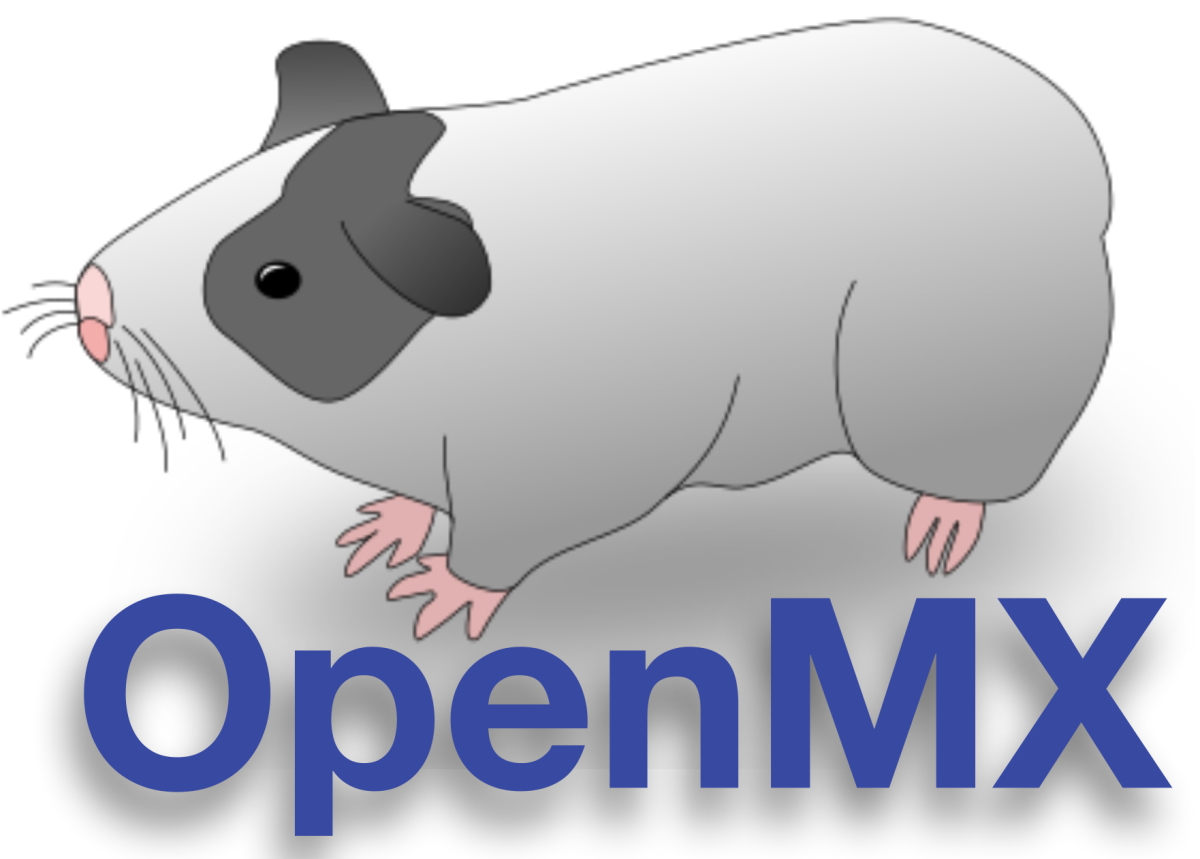
The OpenMx Project. Funded by NIH, OpenMx is a software development project for an open source Structural Equation Modeling (SEM) package that is free of charge and tied into the R statistical system. The OpenMx project involves collaborators at the University of Virginia, Medical College of Virginia, University of Chicago, University of Houston, McMaster University and University of Edinburgh. The project was officially released in the fall of 2010 and has been downloaded more than 70,000 times. Statistical researchers, quantitative psychologists, and software developers who may be thinking about writing extensions to SEM are encouraged to visit the OpenMx Wiki where they can learn about the project and possibly either contribute to the project or find software and open source code that may help them in their own work.
EGAnet: Exploratory Graph Analysis: A Framework for Estimating the Number of Dimensions in Multivariate Data Using Network Psychometrics
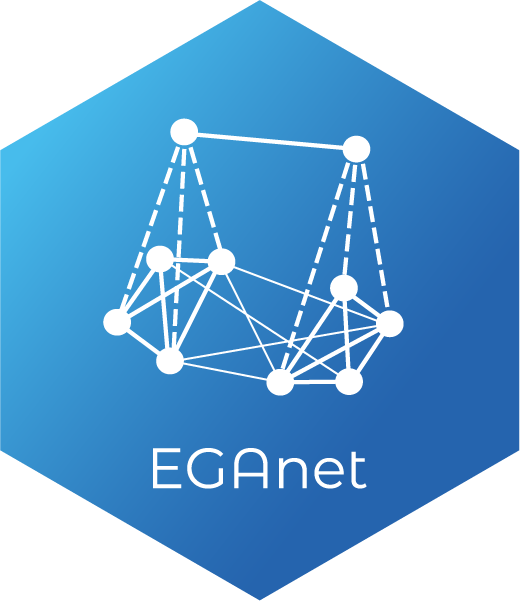
An implementation of the Exploratory Graph Analysis (EGA) framework for dimensionality assessment. EGA is part of a new area called network psychometrics that focuses on the estimation of undirected network models in psychological datasets. EGA estimates the number of dimensions or factors using graphical lasso or Triangulated Maximally Filtered Graph (TMFG) and a weighted network community analysis. A bootstrap method for verifying the stability of the estimation is also available. The fit of the structure suggested by EGA can be verified using confirmatory factor analysis and a direct way to convert the EGA structure to a confirmatory factor model is also implemented. Documentation and examples are available.
transforEmotion: Sentiment Analysis for Text, Image and Video Using Transformer Models
With transforEmotion you can use cutting-edge AI/transformer models for zero-shot emotion classification of text, image, and video in R, all without the need for a GPU, subscriptions, paid services, or using Python. All data is processed locally on your machine, and nothing is sent to any external server or third-party service. This ensures full privacy for your data.
https://github.com/atomashevic/transforEmotion
https://cran.r-project.org/web/packages/transforEmotion/index.html
latentFactoR: Data Simulation Based on Latent Factors
Generates data based on latent factor models. Data can be continuous, polytomous, dichotomous, or mixed. Skews, cross-loadings, wording effects, population errors, and local dependencies can be added. All parameters can be manipulated
https://cran.r-project.org/web/packages/latentFactoR/index.html
AI-GENIE: Generative Psychometrics with Automatic Item Generation and Validation via Network-Integrated Evaluation
The rapid advancement of artificial intelligence (AI), particularly large language models (LLMs), has introduced powerful tools for various research domains, including psychological scale development. This study presents a fully automated method to efficiently generate and select high-quality, non-redundant items for psychological assessments using LLMs and network psychometrics. Our approach called, Automatic Item Generation and Validation via Network-Integrated Evaluation (AI-GENIE), reduces reliance on expert intervention by integrating generative AI with the latest network psychometric techniques. The efficacy of AI-GENIE was evaluated through Monte Carlo simulations using the Mixtral, Gemma 2, Llama 3, GPT 3.5, and GPT 4o models to generate item pools that mimic Big Five personality assessment. The results demonstrated improvement in item selection efficiency, with overall average increases of 9.78-17.80 in normalized mutual information in the final item pool across all models. After, each model in AI-GENIE generated a Big Five inventory that was administered to independent, representative samples (N = 1000 each) in the U.S. The empirical results show that the items produced across all models were diverse, theoretically consistent, and structurally stable. Taken together, these findings demonstrate that AI-GENIE is a highly effective tool to automate and streamline scale development and validation process.
Package to be released soon.
Pre-print here: https://osf.io/preprints/psyarxiv/fgbj4
The Design and Data Analytics Seminar Series
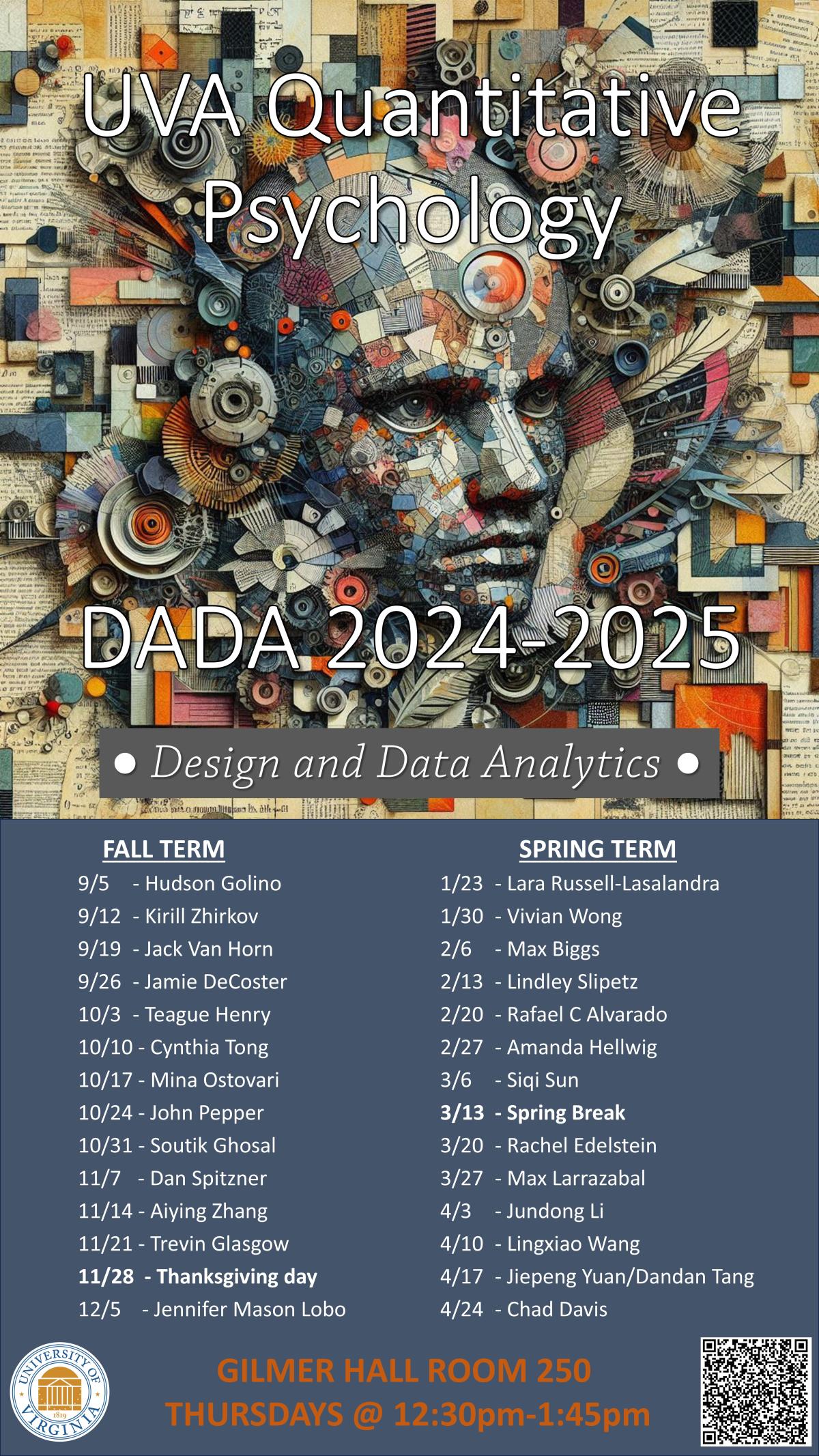
The Design and Data Analytics Seminar Series is a dynamic, student-led initiative in the UVA Department of Psychology that fosters intellectual engagement and interdisciplinary collaboration in the realm of quantitative psychology. Affectionately referred to as “DADA”, this seminar series provides a platform for faculty, graduate students, postdoctoral fellows, and external scholars to share their expertise and insights on both theoretical and applied quantitative approaches in data analysis, modeling, and inference.
DADA covers a broad spectrum of topics, ranging from foundational statistical methods and cutting-edge machine learning techniques to innovative applications in diverse domains such as psychology, healthcare, neuroscience, social sciences, etc. Each session is designed to facilitate meaningful discussions, offering attendees an opportunity to explore novel methodologies, critically engage with research findings, and build connections across disciplines.
By creating a welcoming and interactive environment, the DADA Seminar Series enables students and researchers to stay at the forefront of advancements in data-driven discovery, sharpening their analytical skills while fostering a vibrant, collaborative community dedicated to solving complex real-world problems in the brain and psychological sciences.
Putting a Doctorate in Quantitative Psychology to Work for You!
A doctoral degree in Quantitative Psychology from UVA opens doors to a range of career opportunities in academia, government, industry, and healthcare. Our graduates are well-suited for roles as university professors, teaching and conducting research in psychology and advanced statistical methods. In research settings, they contribute as methodologists, designing experiments and developing innovative approaches to analyze complex behavioral data. In government and industry, they are sought after as psychometricians, data scientists, or consultants, applying their expertise to areas like public policy, human resources, marketing, and healthcare analytics. Additionally, they play critical roles in improving psychological assessments, advancing computational models of human behavior, and developing evidence-based solutions to real-world problems. Their skills are particularly valued on interdisciplinary research teams, where understanding both human behavior and data analysis is essential.